
The Dst index can be used to identify the three phases of these storms: the initial phase, the main phase and the recovery phase. The most important types of these dynamics are geomagnetic storms. Most often, the Dst is used as a measure of the strength of the axi-symmetric magnetosphere currents, capturing the dynamics of the inner magnetospheric current system. The index, introduced by Sugiura (1963), is the average of the magnetic disturbance of the Earth's magnetic field horizontal component. The disturbance storm time (Dst) index is calculated from the measurements of four ground-based magnetometer stations, located close to the equator and spread evenly across the Earth ( Sugiura and Kamei, 1991). Finally, different methodologies for training the neural network are explored in order to remove the persistence behavior from the results. The new measure, based on Dynamical Time Warping, is capable of identifying results made by the persistence model and shows promising results in confirming the visual observations of the neural network's output.
#Omniweb dst series#
In this work, a new method is proposed to measure whether two time series are shifted in time with respect to each other, such as the persistence model output versus the observation. However, visual inspection showed that the predictions made by the neural network were behaving similarly to the persistence model. Inspection of the model's results with the correlation coefficient and RMSE indicated a performance comparable to the latest publications. To show where the classical metrics are lacking, we trained a neural network, using a long short-term memory network, to make a forecast of the disturbance storm time index at origin time t with a forecasting horizon of 1 up to 6 h, trained on OMNIWeb data. However, these classical metrics sometimes fail to capture crucial behavior. These models are evaluated with metrics such as the root-mean-square error (RMSE) and Pearson correlation coefficient.
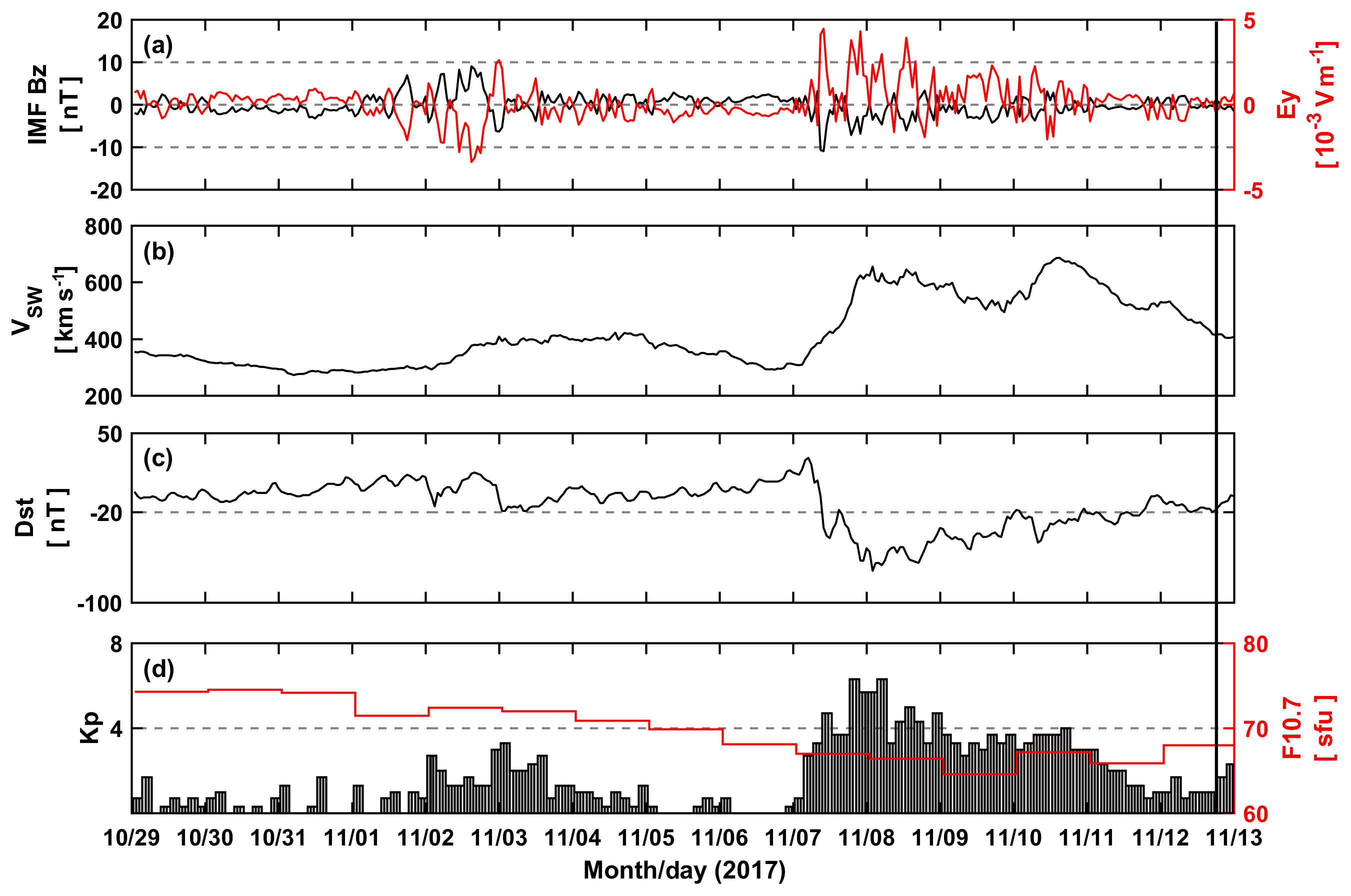

In particular, the forecasting of geomagnetic indices with neural network models is becoming a popular field of study. Models based on neural networks and machine learning are seeing a rise in popularity in space physics.
